The fast-paced world of Forex, commodities, cryptocurrencies, and other financial assets trading and investing requires access to massive amounts of data. Investors and traders must process this data to anticipate market movements and make informed decisions. Artificial Intelligence (AI) has revolutionized this process, gathering data from a broad range of sources—including traditional financial data, economic reports, and social media sentiment—and feeding this into predictive models that generate actionable insights. This article explores how AI gathers and organizes this data to help traders and investors make accurate predictions across different markets.
How AI collects and processes large volumes of data
AI excels in gathering and processing data from a wide range of sources, each offering unique insights into market conditions. By collecting real-time financial data, scraping economic reports, and monitoring social media sentiment, AI-powered systems create a comprehensive view of the factors influencing Forex, commodities, cryptocurrencies, bonds, and stock markets.
Below are examples that demonstrate how AI processes data from these markets to help traders make accurate predictions.
Forex data
In Forex trading, AI models rely on real-time exchange rates, economic indicators like inflation, GDP, and central bank interest rate decisions to predict currency movements.
- Example: Bank of England rate hike in 2023
In 2023, the Bank of England increased interest rates to combat persistent inflation. AI systems tracked the announcement in real time, processing economic data alongside social media sentiment to evaluate its impact on the GBP/USD pair. Traders using AI-driven insights were able to adjust their positions promptly, benefiting from the currency’s movement as the market reacted to the news.
Commodities data
Commodity prices are influenced by supply and demand dynamics, geopolitical events, and weather conditions. AI systems track all these data points to help traders anticipate market shifts.
- Example: European energy crisis and Gas prices in 2023
During the European energy crisis in 2023, geopolitical tensions and sanctions caused significant disruptions in the energy market. AI systems scraped news sources and government reports while monitoring supply constraints, helping traders predict the effect on natural gas prices. AI-powered models alerted traders to price spikes, allowing them to adjust their positions and capitalize on the volatility in energy contracts.
Cryptocurrency data: Social media sentiment and on-chain metrics
Cryptocurrencies are highly volatile, influenced by social media sentiment, technological advancements, and on-chain metrics. AI systems gather and analyze these data points to predict short-term price movements in the crypto markets.
- Example: Ethereum price surge during Shanghai upgrade in 2023
AI systems monitored discussions on Twitter and Reddit about the Ethereum Shanghai upgrade in 2023. By analyzing social media sentiment and blockchain metrics, AI models forecasted a significant price surge in Ethereum. Traders relying on these AI-driven insights positioned themselves early, benefiting from the price increase following the upgrade.
Bond market data: Interest rate announcements and inflation reports
AI systems in bond markets process inflation reports, interest rate changes, and central bank policies. These inputs help AI models predict shifts in bond yields and provide insights on bond portfolio management.
- Example: US treasury yields in 2023
In mid-2023, U.S. Treasury yields were influenced by rising inflation and the Federal Reserve’s policy decisions. AI systems scraped central bank reports and processed macroeconomic data to predict changes in the yield curve. Bond traders using these AI-driven models adjusted their portfolios, mitigating interest rate risks and capturing gains as yields fluctuated.
Stock market data: Earnings reports and market sentiment
Stock prices are affected by a combination of earnings reports, economic data, news, and market sentiment. AI systems track these factors, analyzing both fundamental and sentiment-driven indicators to predict stock price movements.
- Example: Nvidia’s stock surge in 2023 due to AI hype
In 2023, Nvidia’s stock saw rapid price appreciation due to the rising demand for AI chips. AI systems analyzed earnings reports, news coverage, and social media sentiment, predicting strong upward movements. Investors using these AI-powered insights were able to capitalize on Nvidia’s stock rally, positioning themselves ahead of the market.
AI's role in aggregating and processing data from diverse sources
AI’s ability to collect and process vast amounts of data from financial markets allows traders to stay ahead of market movements. AI systems collect real-time data from financial providers, scrape news and economic reports, and analyze social media sentiment.
Below are real-world examples that demonstrate how AI processes data in various markets.
- Real-time data feeds from financial providers
AI systems leverage APIs from financial data providers like Bloomberg and Refinitiv to access up-to-the-minute information on asset prices, interest rates, bond yields, and commodity prices. This real-time data is crucial for traders who need to respond swiftly to market changes.
- Example: Gold price surge in early 2023
AI systems tracked the rise in gold prices in early 2023 as global economic uncertainty increased. Real-time data feeds from commodity exchanges allowed AI models to alert traders about the price surge, enabling them to adjust their positions and capitalize on short-term opportunities in the gold market.
- Web scraping for news and economic indicators
AI systems use web scraping tools to extract critical information from central bank websites, government portals, financial news outlets, and economic reports. These sources provide data on interest rates, inflation, and other macroeconomic indicators that influence Forex, bonds, and stock markets.
- Example: US Federal Reserve’s rate hikes in 2023
In 2023, AI systems tracked U.S. Federal Reserve interest rate announcements and processed inflation data in real-time. These insights helped bond traders predict yield curve shifts and adjust their portfolios, as bond prices typically fall when rates rise.
- Social media sentiment analysis
AI-powered Natural Language Processing (NLP) tools monitor social media platforms like Twitter, Reddit, and LinkedIn, as well as financial forums. These systems analyze sentiment shifts and emerging trends, which can significantly impact stock prices, commodities, and cryptocurrencies.
- Example: Meme stocks and social media influence in 2023
In 2023, AI systems tracked social media platforms like Reddit’s r/WallStreetBets to monitor discussions about meme stocks. Companies like AMC Entertainment experienced significant price movements based on social media sentiment. AI models analyzed these conversations and provided traders with early alerts, allowing them to profit from the volatility.
Data cleaning
AI doesn’t just gather data—it also ensures that the information is accurate and reliable. Data cleaning is one of the most crucial steps in AI's ability to provide accurate and reliable insights for traders and investors. When AI collects data from multiple sources such as financial markets, social media platforms, government reports, and news outlets, the raw data is often messy, inconsistent, or incomplete. Without proper data cleaning, this "dirty" data can lead to inaccurate models and poor trading decisions. AI systems apply a range of techniques to clean the data, ensuring that it is usable and reliable for predictive models across various markets like Forex, commodities, bonds, and cryptocurrencies.
Here’s how AI cleans data:
1. Removing errors and duplicates
Raw data often contains errors or duplicate entries that can distort analysis. AI systems automatically detect these issues and eliminate them to maintain the accuracy of the dataset.
- Example: Forex trading
In the Forex market, price feeds may occasionally contain incorrect data points due to technical glitches or anomalies during times of extreme volatility. For instance, during a flash crash, sudden extreme price fluctuations may appear that don’t reflect the real market conditions. AI identifies these outliers as errors and removes them to ensure the model isn’t skewed by incorrect data.
2. Filling in missing data
Incomplete data is common, especially when scraping information from different sources. AI systems use historical data, statistical techniques, and machine learning to intelligently fill in gaps.
- Example: Bond markets
In bond markets, certain economic data, such as GDP or employment statistics, may not be released consistently across all regions or time periods. AI uses historical data and interpolates values to fill in missing information. For instance, if a particular inflation rate report is delayed, AI systems can use trends from previous periods and similar economic indicators to estimate the missing data, ensuring continuity in analysis.
3. Detecting and correcting anomalies
Anomalies in data can occur due to various reasons, including reporting errors, market manipulation, or sudden unforeseen events. AI is capable of identifying these anomalies and correcting them.
-
Example: Stock market
In the stock market, a sudden spike in trading volume or price due to incorrect trading algorithms or "fat-finger" errors can create data anomalies. AI systems can detect these abnormal spikes by comparing them to historical norms and correct them before they feed into the predictive models, preventing faulty predictions and trading errors.
4. Converting unstructured data into usable formats
Many data sources, especially from social media and news outlets, come in unstructured formats—text, images, or videos—that aren’t directly analyzable by traditional models. AI processes this data, extracting key information and converting it into structured formats for further analysis.
- Example: Cryptocurrency markets and Social Media
Cryptocurrencies are often influenced by public sentiment on platforms like Twitter or Reddit. For instance, during the 2023 meme coin craze, AI systems monitored thousands of posts and discussions about specific coins. AI’s Natural Language Processing (NLP) tools extracted sentiment (positive, neutral, negative) and converted the text into structured data, which could then be fed into models predicting price movements. This structured data allows the system to quantify sentiment-driven volatility more accurately.
5. Cross-referencing data for accuracy
To ensure accuracy, AI systems cross-reference data from multiple sources. If the same data point is collected from two or more reputable sources, it is considered reliable. If discrepancies are found, AI will flag the data for review or exclude it from the model.
- Example: Commodities markets
In the commodities market, supply and demand information might come from various global sources, each offering different figures. For example, oil production reports from OPEC and independent energy organizations might differ slightly. AI cross-references these sources, identifying the most reliable data to ensure that traders make decisions based on accurate information about global supply levels.
6. Normalizing data for consistency
Data collected from multiple sources often comes in different formats or units, which can lead to inconsistencies. AI systems normalize the data, ensuring it follows a uniform format, making it easier to analyze.
- Example: Global forex markets
Exchange rates and economic indicators from different countries might be reported in various formats or units. AI normalizes this data, converting currencies into a base format (like USD), ensuring consistency across all inputs. This normalization allows AI models to compare currency pairs or economic performance across countries with ease.
AI’s role in feeding predictive models
Once AI systems have gathered and cleaned the data, it feeds it into predictive models. AI’s ability to feed vast amounts of data into predictive models is one of its most powerful applications in financial markets. These AI-driven models can analyze multiple data sources—ranging from economic indicators and social media sentiment to real-time market data—and generate insights that inform trading decisions.
- Forex: Predicting currency movements
AI models in Forex predict currency fluctuations by analyzing macroeconomic data, central bank decisions, and geopolitical events.
- Example: Euro/USD predictions during the 2023 economic slowdown
In 2023, AI models analyzed economic indicators from the eurozone, including inflation data and employment reports, to predict movements in the EUR/USD currency pair. Traders who used these models were able to anticipate currency shifts and adjust their strategies accordingly.
- Commodities: Forecasting supply chain disruptions
AI models forecast commodity price changes by processing data related to supply chain disruptions, geopolitical events, and weather conditions.
- Example: Lithium price spike in 2023
As demand for electric vehicles surged in 2023, AI systems analyzed disruptions in lithium mining operations and supply constraints. By feeding these data points into predictive models, AI forecasted a rise in lithium prices, allowing traders to profit by taking early positions in the market.
- Bonds: Anticipating yield curve changes
In bond markets, AI predictive models analyze inflation reports, interest rate announcements, and investor sentiment to anticipate changes in bond yields.
- Example: US Treasury yield forecasts in 2023
In 2023, AI models accurately predicted U.S. Treasury yield changes based on inflation trends and Federal Reserve policy. Investors using AI models were able to mitigate interest rate risks by adjusting bond portfolios ahead of the market.
- Stocks: Predicting price movements based on market sentiment
In stock markets, AI predictive models combine fundamental analysis and sentiment analysis to anticipate stock price movements.
- Example: Tesla stock fluctuations in 2023
In 2023, AI models analyzed earnings reports and social media discussions about Tesla. By predicting short-term stock price fluctuations, traders using AI-driven insights were able to hedge against potential losses and capitalize on price surges.
- Cryptocurrencies: Predicting sentiment-driven volatility
In the cryptocurrency market, AI predictive models analyze social media sentiment, blockchain activity, and macroeconomic data to forecast price movements.
- Example: Ethereum rally in 2023
AI systems predicted Ethereum’s price rally in 2023 by analyzing blockchain metrics and social media sentiment around the network upgrade. Traders using these AI models were able to take early positions before Ethereum's price surged.
Conclusion
AI has become an indispensable tool in the fast-paced world of Forex, commodities, bonds, stocks, and cryptocurrency trading. By gathering vast amounts of data from real-time market feeds, economic reports, and social media sentiment, AI empowers traders and investors to make informed, timely decisions. AI-driven predictive models enable traders to anticipate market movements with greater accuracy, helping them navigate the complexities of global financial markets.Data cleaning is a vital part of AI’s process, ensuring that the information fed into predictive models is accurate, reliable, and free from inconsistencies.
As AI technology continues to evolve, its role in financial markets will become even more prominent, giving traders the competitive edge they need to succeed in today’s volatile and dynamic markets.
CFDs are complex instruments and come with a high risk of losing money rapidly due to leverage. You should consider whether you understand how CFDs work and whether you can afford to take the high risk of losing your money. The Article/Information available on this website is for informational purposes only, you should not construe any such information or other material as investment advice or any other research recommendation. Nothing contained on this Article/ Information in this website constitutes a solicitation, recommendation, endorsement, or offer by LegacyFX and A.N. ALLNEW INVESTMENTS LIMITED in Cyprus or any affiliate Company, XE PRIME VENTURES LTD in Cayman Islands, AN All New Investments BY LLC in Belarus and AN All New Investments (VA) Ltd in Vanuatu to buy or sell any securities or other financial instruments in this or in in any other jurisdiction in which such solicitation or offer would be unlawful under the securities laws of such jurisdiction. LegacyFX and A.N. ALLNEW INVESTMENTS LIMITED in Cyprus or any affiliate Company, XE PRIME VENTURES LTD in Cayman Islands, AN All New Investments BY LLC in Belarus and AN All New Investments (VA) Ltd in Vanuatu are not liable for any possible claim for damages arising from any decision you make based on information or other Content made available to you through the website, but investors themselves assume the sole responsibility of evaluating the merits and risks associated with the use of any information or other Article/ Information on the website before making any decisions based on such information or other Article.
Editors’ Picks
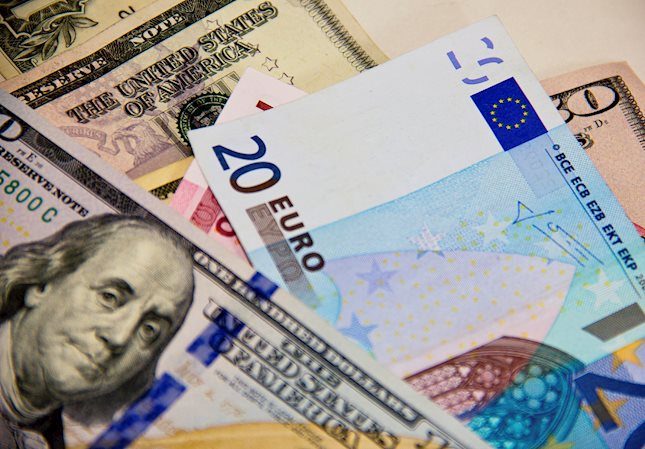
EUR/USD extends recovery beyond 1.0400 amid Wall Street's turnaround
EUR/USD extends its recovery beyond 1.0400, helped by the better performance of Wall Street and softer-than-anticipated United States PCE inflation. Profit-taking ahead of the winter holidays also takes its toll.
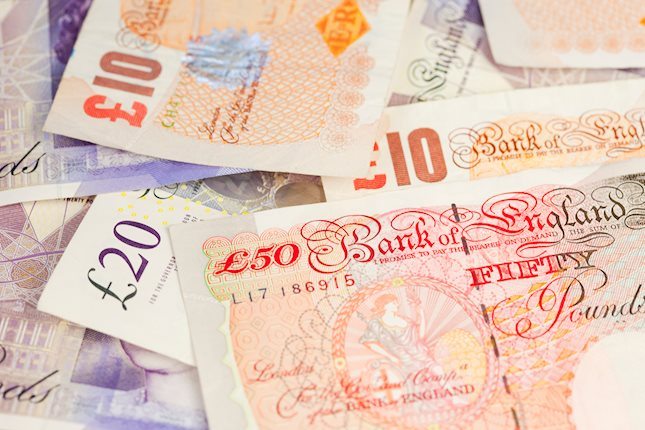
GBP/USD nears 1.2600 on renewed USD weakness
GBP/USD extends its rebound from multi-month lows and approaches 1.2600. The US Dollar stays on the back foot after softer-than-expected PCE inflation data, helping the pair edge higher. Nevertheless, GBP/USD remains on track to end the week in negative territory.
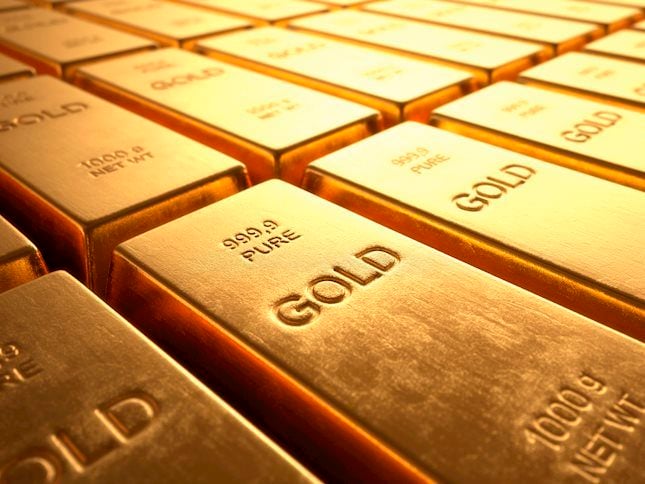
Gold rises above $2,620 as US yields edge lower
Gold extends its daily rebound and trades above $2,620 on Friday. The benchmark 10-year US Treasury bond yield declines toward 4.5% following the PCE inflation data for November, helping XAU/USD stretch higher in the American session.
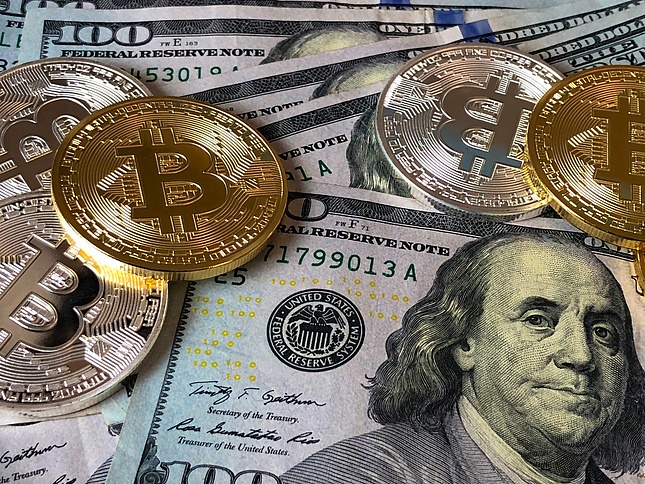
Bitcoin crashes to $96,000, altcoins bleed: Top trades for sidelined buyers
Bitcoin (BTC) slipped under the $100,000 milestone and touched the $96,000 level briefly on Friday, a sharp decline that has also hit hard prices of other altcoins and particularly meme coins.
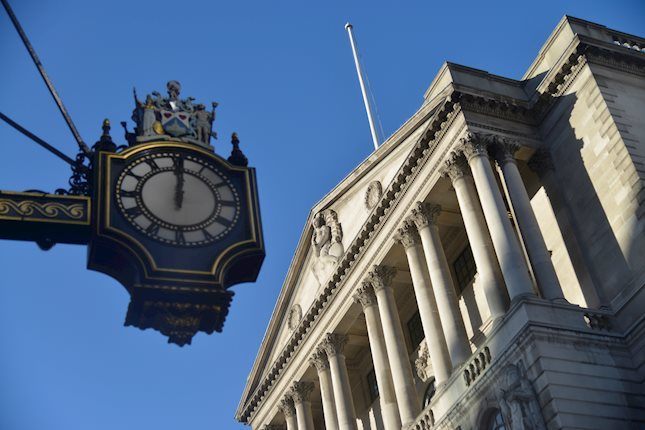
Bank of England stays on hold, but a dovish front is building
Bank of England rates were maintained at 4.75% today, in line with expectations. However, the 6-3 vote split sent a moderately dovish signal to markets, prompting some dovish repricing and a weaker pound. We remain more dovish than market pricing for 2025.
RECOMMENDED LESSONS
Making money in forex is easy if you know how the bankers trade!
Discover how to make money in forex is easy if you know how the bankers trade!
5 Forex News Events You Need To Know
In the fast moving world of currency markets, it is extremely important for new traders to know the list of important forex news...
Top 10 Chart Patterns Every Trader Should Know
Chart patterns are one of the most effective trading tools for a trader. They are pure price-action, and form on the basis of underlying buying and...
7 Ways to Avoid Forex Scams
The forex industry is recently seeing more and more scams. Here are 7 ways to avoid losing your money in such scams: Forex scams are becoming frequent. Michael Greenberg reports on luxurious expenses, including a submarine bought from the money taken from forex traders. Here’s another report of a forex fraud. So, how can we avoid falling in such forex scams?
What Are the 10 Fatal Mistakes Traders Make
Trading is exciting. Trading is hard. Trading is extremely hard. Some say that it takes more than 10,000 hours to master. Others believe that trading is the way to quick riches. They might be both wrong. What is important to know that no matter how experienced you are, mistakes will be part of the trading process.
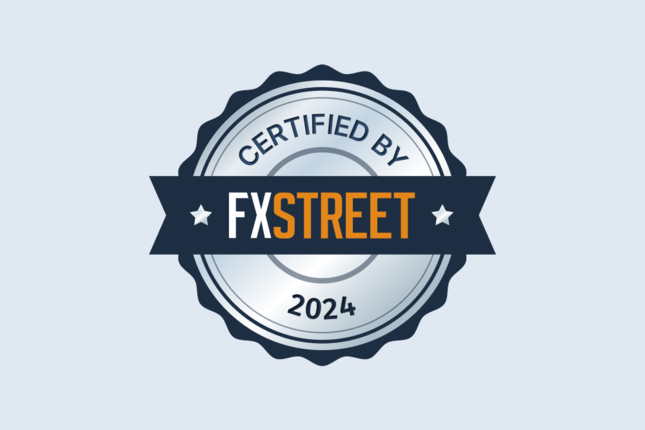
Best Forex Brokers with Low Spreads
VERIFIED Low spreads are crucial for reducing trading costs. Explore top Forex brokers offering competitive spreads and high leverage. Compare options for EUR/USD, GBP/USD, USD/JPY, and Gold.