Machine learning pricing models a guide for investors and traders
|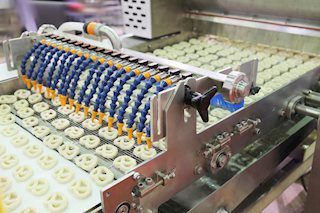
Machine Learning (ML) has become a game-changer in the financial markets, particularly in the realm of pricing models. For investors and traders, understanding how ML-based models function can offer a significant edge, helping to make more informed decisions. This article explains the key ML pricing models, their applications, and the potential benefits they bring to trading and investing.
Understanding machine learning pricing models
Machine Learning models utilize algorithms that learn from historical data to make predictions about future prices.
These models can process vast datasets, including market trends, economic indicators, and news sentiment, to provide more accurate pricing. Unlike traditional models, ML models are adaptive, continuously learning from new data, making them ideal for fast-moving markets like currencies and commodities.
Key machine learning models in pricing
Decision trees and random forests
-
What They Are: Decision Trees are models that split data into branches based on decision rules, helping to identify factors influencing asset prices. Random Forests combine multiple decision trees to improve prediction accuracy.
-
Real-world examples
-
Investment Firms use Random Forests for bond pricing and credit risk assessment. The model considers various factors like interest rates, credit spreads, and market sentiment to price bonds accurately, especially during volatile market conditions.
-
They also use Decision Trees to predict currency price movements, analyzing data from macroeconomic indicators, interest rates, and geopolitical events. This approach helps traders identify opportunities in the FX market, such as anticipating central bank actions or economic releases.
-
Why They Matter: These models are easy to understand and interpret, making them useful for identifying key price drivers. They work well with structured data, such as historical prices and financial ratios.
-
Gradient Boosting Machines (GBM) and XGBoost
-
What they are: These are advanced models that improve upon traditional decision trees by focusing on errors made in previous predictions. Each new tree is built to correct mistakes from the last, enhancing overall model performance.
-
Real-world examples
-
Citadel Securities, a leading market maker, utilizes XGBoost for pricing options and predicting equity prices. The model’s ability to handle large datasets allows Citadel to maintain tight spreads and high-frequency trading strategies.
-
JPMorgan Chase uses Gradient Boosting Machines (GBM) to predict gold prices by analyzing market data and geopolitical risks, accurately forecasting price surges. This approach helps the trading desk adjust positions and capture profitable opportunities.
-
- Why they matter: GBM and XGBoost are highly accurate and robust, making them popular for complex pricing tasks like predicting bond yields or equity prices. They are especially good at handling non-linear relationships and large datasets.
Neural networks and deep learning
-
What they are: Neural Networks are inspired by the human brain’s structure and are designed to recognize complex patterns in data. Deep Learning models, a subset of Neural Networks, involve multiple layers that allow them to capture intricate data relationships.
-
Real-world examples
-
Goldman Sachs employs Neural Networks to price exotic options and complex derivatives, such as those linked to multiple underlying assets or with path dependencies. By analyzing extensive historical data, these models offer more accurate pricing than traditional methods like Black-Scholes.
-
Shell uses Deep Learning models to forecast oil prices by analyzing market trends, refinery outputs, and inventory levels, enabling more accurate pricing and better-informed hedging strategies.
-
- Why they matter: These models excel in pricing complex assets like options and derivatives, where traditional methods may struggle. They can learn from vast amounts of data, including historical prices, market sentiment, and even macroeconomic variables.
Support Vector Machines (SVM)
-
What they are: SVMs are models that classify data into different categories, often used to identify trends or predict price direction. In pricing, they can help determine whether an asset is likely to go up or down.
-
Real-world examples
-
Morgan Stanley uses SVMs for intraday stock price prediction. This approach helps in developing trading strategies that identify short-term price movements based on historical price patterns and market data.
-
HSBC utilizes SVMs to predict currency price trends, particularly in emerging markets. This helps the bank’s trading desk identify potential shifts in currency pairs where market information is less transparent.
-
- Why they matter: SVMs are powerful when dealing with smaller datasets and can be particularly useful in identifying price movements during less liquid or niche markets.
Reinforcement Learning (RL)
-
What they are: RL models learn by interacting with market environment, such as a financial market, and receiving feedback in the form of rewards or penalties. They adjust strategies dynamically to improve performance over time.
-
Real-world examples
-
DeepMind, owned by Alphabet, has partnered with financial firms to develop RL-based models for pricing energy derivatives. The models adapt in real time, responding to market changes, regulatory shifts, and supply-demand dynamics, allowing for better pricing and risk management.
-
Refinitiv, a data analytics provider, uses RL models for commodity trading strategies. These models adapt in real time to market conditions, optimizing trade execution in volatile markets like oil and metals.
-
- Why they matter: RL is particularly useful in dynamic trading strategies and pricing complex derivatives where real-time adjustments are crucial.
Advantages of ML pricing models
-
Adaptability: Unlike traditional models, ML models can adapt quickly to new data, ensuring that pricing remains relevant even in rapidly changing markets.
-
Improved accuracy: By analyzing complex and large datasets, ML models often provide more accurate price predictions, helping traders identify better entry and exit points.
-
Automation: ML models can automate the pricing process, reducing the time spent on manual analysis and allowing for quicker decision-making.
-
Risk mitigation: Through advanced scenario analysis, these models help investors assess potential risks and make informed adjustments to their portfolios.
Challenges to consider
While ML pricing models offer numerous benefits, it’s important to acknowledge some challenges:
-
Data quality: The accuracy of ML models heavily depends on the quality of data input. Inconsistent or poor-quality data can lead to inaccurate predictions.
-
Model transparency: Some ML models, especially Neural Networks, are often seen as “black boxes,” making it difficult to understand how they arrive at their predictions.
-
Overfitting: ML models can sometimes overfit historical data, meaning they perform well on past data but struggle to predict future outcomes accurately. Regular validation and testing are necessary to mitigate this risk.
Machine Learning pricing models are redefining the way investors and traders approach valuation and market analysis. By offering more accurate, adaptable, and comprehensive pricing solutions, ML models empower market participants to make better-informed decisions. However, successful implementation requires a keen understanding of the models, ongoing validation, and a robust approach to data management.
As these models continue to evolve, they will undoubtedly play an increasingly critical role in the future of financial markets. By embracing ML-driven pricing techniques, investors and traders can gain a competitive edge in today’s complex and rapidly evolving markets.
Information on these pages contains forward-looking statements that involve risks and uncertainties. Markets and instruments profiled on this page are for informational purposes only and should not in any way come across as a recommendation to buy or sell in these assets. You should do your own thorough research before making any investment decisions. FXStreet does not in any way guarantee that this information is free from mistakes, errors, or material misstatements. It also does not guarantee that this information is of a timely nature. Investing in Open Markets involves a great deal of risk, including the loss of all or a portion of your investment, as well as emotional distress. All risks, losses and costs associated with investing, including total loss of principal, are your responsibility. The views and opinions expressed in this article are those of the authors and do not necessarily reflect the official policy or position of FXStreet nor its advertisers.